For several years at Emergence, we've been using what we initially called our "Delta Metric" to evaluate how effectively AI software learns from users and evolves over time. For the first time, we are sharing this metric publicly. We call it the AI Emergence Rate.
The AI Emergence Rate measures the impact of AI software through the incorporation of proprietary business data and processes, leading to ever-improving outcomes for the software’s users.
In a world where B2B software must improve every day, the AI Emergence Rate metric is what separates stagnant AI software startups from those that continuously optimize their solutions. The result is better outcomes for customers and better chances of startup’s success in an incredibly competitive AI software landscape.
.png)
Why We Built the Emergence Rate
Our journey to the Emergence Rate began with our AI Coaching Networks thesis in 2017. Over years of diligence, we observed how the best enterprise AI software startups responded to the constantly evolving needs of their customers.
By today’s measures, even the best startups of the last two decades operated relatively statically. This is simply a reflection of real-world constraints: the number of engineers they can employ, the time it takes to turn feature requests into code, the technological constraints of this era of software, and their customers’ discomfort with too many big changes confusing their users.
In the Client-Server Era, a slick demo and out-of-the-box performance typically won prospects over. Companies paid big upfront license fees for on-prem installations. Meaningful upgrades occurred, it seemed, whenever the Olympic Games came back around. Over time, they struggled to keep up with their customers’ evolving needs. Then a new generation of SaaS startups, led by Salesforce.com, emerged to address new challenges, and customers migrated to them.
The cycle has now repeated with the generative AI breakthrough. The new era of AI software has unleashed unprecedented capabilities that can help startups break this cycle and add value for customers over the long haul. The new leaders will be AI software startups that continuously increase the value of their customers’ proprietary assets–most importantly, the ingenuity of their workforce.
Here is how both sides of the software marketplace–startups and their customers–can think about the Emergence Rate framework, and measure the revolutionary impact of AI on their businesses:
- For Startups: A high Emergence Rate–the ability for AI models to automatically refine and improve themselves–distinguishes market winners from those who will stagnate. Startups with a high Emergence Rate deliver products that evolve with their customers, commanding premium prices, and avoiding the churn common among early adopters of AI software.
- For Customers: AI Software with a high Emergence Rate becomes increasingly valuable to their business over time, unlike traditional software, which depreciates. This self-improving AI soon becomes the indispensable heart of the company, worth paying for indefinitely, and providing compounding advantages that create massive strategic differentiation in the market.
How We Define the Emergence Rate: Three Key Metrics
.png)
Through our portfolio work, we've refined the Emergence Rate into three essential dimensions:
- Rate of Change: How frequently does an AI system assess variants based on granular user activity? The faster an AI system integrates unique behaviors, the higher its Emergence Rate.
Today's most advanced AI software implements continuous learning loops, whether through ambient observations (e.g., RPA) or user collaborations within agentic workflows. The best AI software captures and applies insights in real-time without requiring manual updates, as models dynamically change with every new piece of user interaction that’s generated.
In many ways, this is what Scale AI and Surge AI provide for foundation models–filling in large-model deficiencies with the unique abilities of humans (the “HF” in RLHF). Startups focusing on proprietary commercial markets have the advantage of workflow relationships with their customers’ users. The large models won’t have access to this “firewalled” user activity. Startups that carefully cultivate this software relationship at the center of user activity, protecting customers’ treasured IP, will become indispensable.
- Quality of Change: Not all variations improve outcomes—and quality matters more than volume. One poor recommendation can undermine trust in an entire system, even if preceded by many good ones.
At Emergence, we've observed that outcome improvements can be identified through rigorous variant testing. Our portfolio companies typically analyze hundreds of interactions, group users into performance tiers, and identify successful patterns. With AI, we now have granular data to test, using interstitial feedback as proxies where feedback loops are long (as in sales cycles).
For example, when a patient receives a more personalized note from a healthcare provider following a visit, they may be more likely to take the recommended actions, which could improve patient adherence.
From an engineering lens, an AI may be able to observe the qualities of a successful pull request (e.g., minimal discussion, no downstream errors, no rollback) and tailor its style and tests to adhere as closely as possible to those attributes.
- Breadth of Adoption: Are insights from top performers disseminated organization-wide? This requires excellent user experience design. Once a successful behavior is identified, the system can either automate the process or guide all users toward it, through dynamic UX.
The goal isn't to create a "mushy middle" of average performance, but to maintain human innovation while elevating the quality of the average outcome. This could mean anything from increasing the rate of successful closes, to raising average ACV, to improving cohort retention rates. Any business scenario can benefit from this cycle.
Finally, keeping human users in the loop throughout enables them to adopt recommendations and experiment with variations, preventing dependency on what we call "Crutch Software"— systems that do the work, but don't help users learn or improve.
How Companies Can Measure Their Own Emergence Rate

Based on broad diligence and portfolio experience, here is a five point framework for a startup to measure its Emergence Rate:
- Track variant identification: Document how many unique user behaviors the software identifies within a given timeframe. The more granular the detection, the better.
- Implement outcome-based metrics: Define clear success metrics for the specific industry or function the software is built for. In sales, this might be deal closure rates; in healthcare, patient outcomes; in life science, clinical trial patient recruitment.
- Assess variant quality: Measure how much performance variance can be attributed to specific behaviors the AI has identified. A number of our AI companies bootstrapped variant quality by first assessing who on the team produced the variant. If that person is a high performer in their area, their actions become a proxy for variant quality.
- Monitor UX evolution: Count the number of automatic UX or agentic workflow changes that occur reflecting learned behaviors. These should increase over time as the system identifies successful patterns.
- Measure adoption speed: Track how quickly users adopt newly recommended behaviors, and whether those behaviors persist over time, or inexplicably fall away.
The most successful companies in our portfolio collect historical user data, analyze patterns across performance tiers, and focus particularly on raising the bar by capturing and sharing the brilliance of outliers across the organization, so that all may benefit.
The Value of Proprietary Data
Every kind of business, no matter its size or activity, is built on unique intellectual property, industry-specific knowledge/processes, and proprietary datasets. This proprietary “data” are what AI software startups can use to help those businesses overcome what we call the AI Plateau.
AI software startups with a high Emergence Rate populate their platforms with these proprietary data in a way that competitors cannot access. This allows a customer to benefit from increases in the number of successful outcomes at a rate not possible in previous eras of software. In turn, as the AI software learns from human-generated insights that are both rare and valuable, an AI software startup’s Emergence Rate increases, allowing it to better process new data. We call this “The Perpetual Algorithm.”
The advantage for an AI software startup lies in becoming the perpetual algorithm for its domain—continuously learning from user patterns and serving those insights back to its customers in a virtuous, ever-improving cycle.
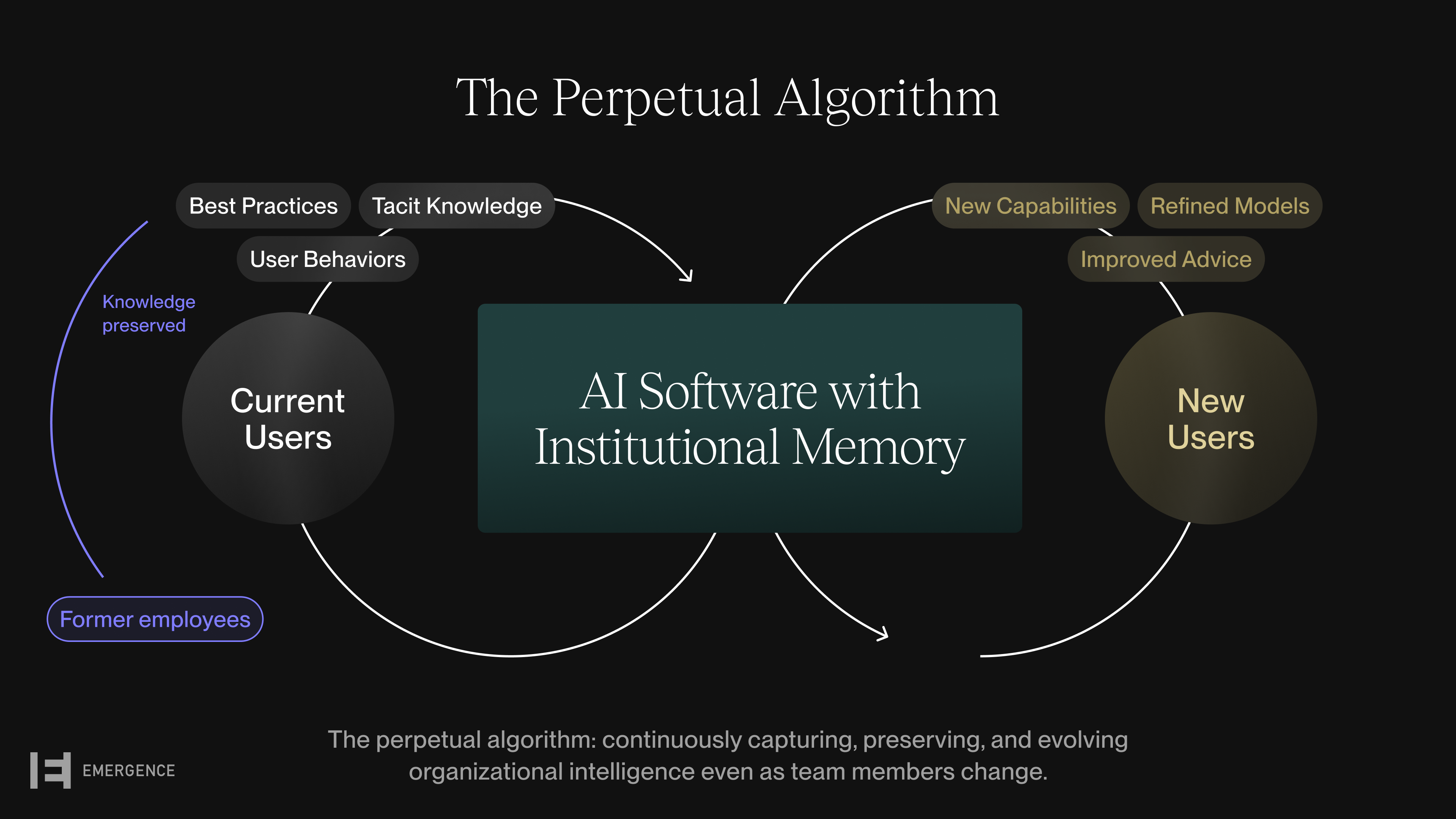
The Future of Adaptive Intelligence
Humans are natural "a-ha engines." Our minds have nearly 100 billion interconnected neurons and store our unique lived experiences, enabling boundless creativity, adaptability, and innovation. We constantly seek breakthroughs that take us beyond the status quo. Improving processes–from the printing press to the tractor to the spreadsheet–is a core way that we express creativity and ingenuity.
The best AI software harnesses this innate human behavior by identifying when users have discovered (or stumbled upon) new successful approaches, validating their impacts on the business, and distributing these insights organization-wide.
Looking ahead, we know AI software with a high Emergence Rate is essential for enterprise success. The most forward-thinking AI software buyers have moved beyond startup-maintained deployments toward constant renewal. Their software evolves continuously based on real-time activity, maintaining their competitive edge by harnessing their proprietary, uniquely-human innovations to improve the entire enterprise. The best startups will be at the center of this new rate of innovation.