Your Success with Generative AI May Come Down to These UX Decisions
A long-time customer support leader we know recently went shopping for a generative AI solution for processing tickets. She shared the framework she was using to evaluate her options, and it came down to three dimensions:
- Cost
- Performance
- UX
Cost and performance were mostly equal, given how much underlying tech all the apps shared. But UX was different. Some companies were doing a much better job at building generative AI capabilities into a more powerful UX and delivering much more value as a result.
Hearing this got us curious about how companies are making UX choices as they integrate generative AI capabilities – and the implications these decisions have for their go-to-market success and the end users they serve. One thing is clear: the most competitive (and likely enduring) companies in the field are the ones that precisely tailor how AI shows up in their products to match user needs and expectations every step of the way. Given the growing commoditization of the underlying tech, we believe thoughtful UX will be the major determinant behind who wins and who loses in this generation of generative AI-enabled applications.
That said, once you start digging into UX options, a ton of questions without obvious answers pop up, including:
- When should a chat interface be utilized?
- How do you teach people how to prompt without adding friction?
- How should co-pilot features be incorporated to get the best out of both the human and the machine?
Our goal with this piece is to give you a handle on all these questions and what good AI UX actually looks like. To do so, we talked to more than a dozen product and design leaders at Notion, Guru, Ironclad, Retool, Maze, and more, who are on the leading edge of all this. What we found was a central tradeoff and lots of hard-won advice.
Trading off flexibility for ease of use
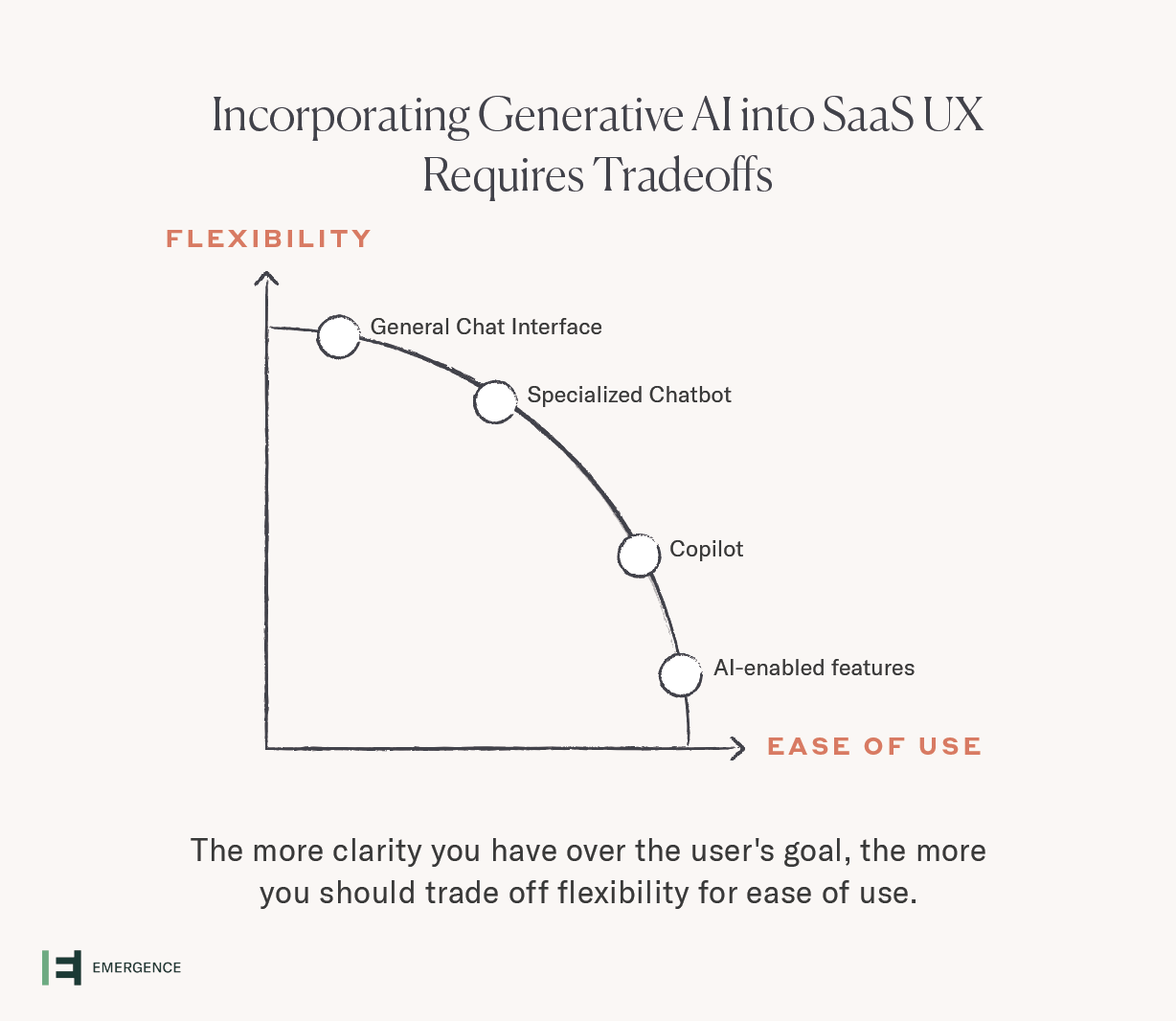
SaaS products have always given users static UX and asked them to point and click their way to their goals. But LLMs break this wide open.
Chat-based interfaces, in particular, give users massive flexibility in the problems they can solve and how they can solve them. At the same time, users may not know what the models can do for them nor how to best prompt them, which adds complexity, friction, and confusion.
Other user interfaces for AI, like co-pilots and embedded features, offer more constraints and ease but trade-off flexibility. They tend to be more useful for more narrowly defined tasks.
The UX designers we spoke to said their UX choices came down to a core question: How much clarity do they have about the user’s goal?
- Whenever you have less clarity, the best practice is to opt for more flexible, general chat interfaces. Not only does this give users more options to choose from, but it also maximizes your learning.
- When you have more clarity and context on user goals, you can move toward more constrained interfaces that make navigation more intuitive and predictable for your user – creating that all-important sense of ease.
One common mistake is picking a single type of UX for your product and feeling like you have to stick with it. Your implementation of AI should take on multiple shapes as you learn more about their goals, as the user moves along their journey to their goal, and even as their goals evolve.
In general, as your user’s goals and the context around them become clearer, designers should narrow their UX to guide folks down a “constrained happy path.” When in doubt, start general and progressively add constraints.
With this in mind, let’s dive deeper into the different types of UX choices that are proving most effective (depending, of course, on the tradeoffs that are right for your product).
General chat: The greeter
Natural language chat is an incredible innovation in UX, but it’s much better for some things than others. It excels in conversational tasks but those requiring more visualization can be confusing to navigate via the blank canvas of chat.
In these instances, general chat is an ideal welcome wagon. It can help you direct folks to the best parts of the product to achieve their goal. They can type their questions, needs, and wants in their own words, and be guided in the right direction without having to click around a complex interface to find their way. At the same time, this UX learns from them so your product gets better at onboarding over time.
General chat presents users with a maze that has almost infinite paths, with lots of entrances and granular destinations. This has its strengths – it can deliver experiences that feel personalized, like they’re meeting the user where they are. However, in B2B software, it’s important for chat to hand off to a more constrained, opinionated UX that gets users where you want them to go. Otherwise, they can get lost or simply feel like it’s taking too long to do what they need to do.

Here are a few best practices when incorporating general chat AI into your product:
- Make your onboarding process more human. This is a great way to make a good first impression on the full variety of users coming in. It can make them feel like they’re getting to know a person, not just a product. It can also reduce the sense of being lost that many users have when being dropped into a point-and-click interface for the first time. Using general chat as a product onboarding tool is something we heard a number of designers talk about with excitement as something they’re experimenting with, but it’s early days in terms of live deployments.
- Collect user data in a natural way. Telling a robot that you’re a marketer is so much more fun than choosing “marketer” from a dropdown menu. The depth and breadth of what humans choose to share with a chatbot is amazing. You can glean so much metadata about them based on how they choose to ask for things or what they say is important to them. You’ll be better able to drop them into the optimal portion of a point-and-click interface, and collect helpful feedback to refine your product development process.
- Deliver answers. In addition to being a helpful onboarding UX, general chat can be helpful to users searching for information. Guru, which provides a knowledge management solution, utilizes a general chat interface to share answers to employee questions. Little context is needed, as the chatbot is trained on company-validated knowledge cards. Chat is an improvement on the already established search box UX for this task.
Remember that if you use general chat to welcome folks into your product, it’s important that it then directs them to more constrained interfaces. It can greet your users, get a sense of what they want, and point them down the road. At their next step, you may need to evolve your UX.
Specialized chat: The usher
As you get a better sense of the user’s goals, designers can add constraints to the chat experience. This can take the form of a specialized chatbot designed to interact with users on a specific topic.
A task-specific bot offers the user clearer bounds for their journey as well as the opportunity to take them further down it. Think of an usher. While the greeter points the user in the direction they should go, the usher walks them to their seat. By constraining the topics for discussion and drawing on a tailored dataset, specialized chat can aid the user on their happy path, trading off flexibility for ease of use.

Here are a few strategies we’ve seen for using specialized chat to give your users more direction without feeling limited.
- Embed goal-specific UX in chat. Monty, a chatbot built by G2, helps their users identify the right software for their needs. Monty asks users a set of interactive questions to guide them to the software vendors they should evaluate. As it learns more, it embeds elements of point-and-click UX in the chat to sharpen the search. When the user arrives at their final vendor list, it links out to G2’s purpose-built evaluation UX to help the user finish the job. Maze is releasing a specialized chatbot embedded within its product testing tool that dynamically chats with the user about their experience with the product prototype, creating an AI-moderated research experience.
- Communicate the chatbot’s limits to your user. Let the user know that the chatbot is focused on a specific goal. A chatbot trained on a specific goal can help limit frustration and calibrate the answers it gives more effectively.
- Give the chatbot a personality. A few designers we spoke with are experimenting with giving the chatbots specific personalities based on the goal they’re focused on. This can help the experience feel more human.
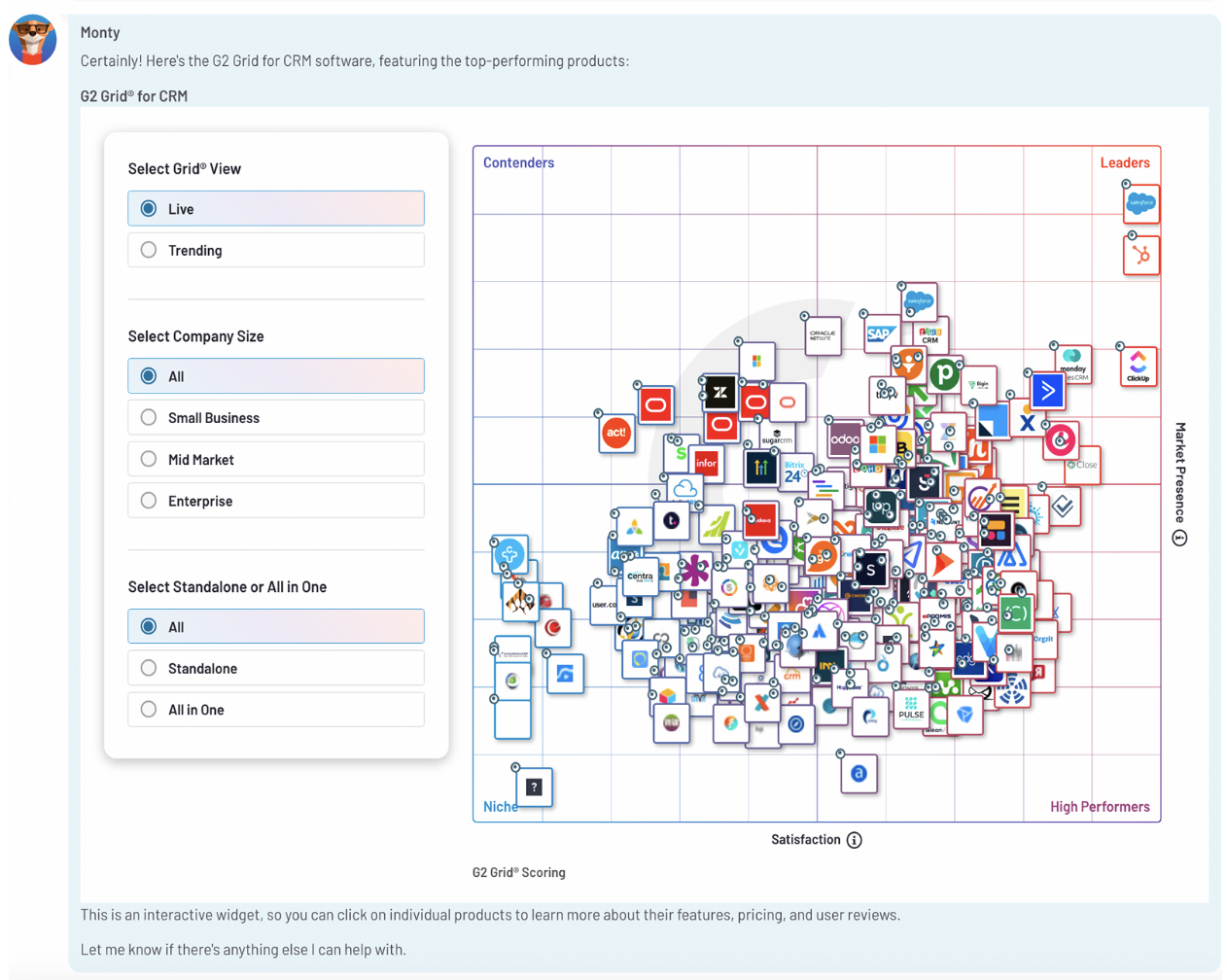
Specialized chatbots come with the same tradeoff between ease and flexibility. Some workflows require in-flow interfaces or UX’s with more visual descriptions not well-suited to chat. More importantly, in most cases, both general and specialized chatbots are reactive instead of proactive. This means that the cognitive burden on the user is still quite high, as is the chance they get lost on their journey. They might need more hand-holding…
Co-pilot: The coach
Enter the co-pilot UX, which you can think of as a coach for your users. A co-pilot further trades-off flexibility to increase ease and reduce a user’s cognitive burden. It embeds into the user’s workflow, enabling it to pop up proactive suggestions along the journey. This integrated approach allows the AI to capture more context, including tying actions to business outcomes. By learning which recommendations a user takes and how those recommendations perform from an outcomes perspective, the AI can improve what it serves up across all users.
At Emergence, we call this an AI Coaching Network and have been investing in co-pilots since 2015, starting with Chorus in sales conversations and Textio in HR writing.

This UX has grown increasingly popular and is now being used in many applications, from coding (Github) to writing (Notion) to contract negotiation (Ironclad).
But it can be a hard UX to design well. On the one hand, poorly built co-pilots can lose user trust and become ignored (or even maligned, think Microsoft Clippy). On the other, overreaching co-pilots run the risk of putting users on autopilot, which could allow AI-generated errors to propagate through business systems. Given the propensity for AI models to hallucinate, active human participation is necessary to ensure trust in these systems for most B2B applications.
To avoid the former, the product and design leaders we spoke to say they’re always closely monitoring confidence thresholds for coaching suggestions made by their co-pilots. They’re starting with fewer, better suggestions and slowly expanding as they gather data on usage and outcomes. Companies like Retool and Textio target “Coaching Acceptance Rates” (the percentage of AI suggestions taken by the user) north of 75% to ensure their co-pilots are trusted.
To avoid building an autopilot instead of a co-pilot, it’s useful to think of the AI/human relationship as an intern/manager relationship. While the intern may produce a first draft, the manager is ultimately responsible for the outcome of the work. An AI co-pilot should be thought of the same way: a way for a worker to learn and accelerate, but ultimately accountability must remain with the manager.
The key to doing this well in your application will be developing UX that encourages people to contribute actively rather than passively. This will ensure accuracy and provide creativity and mutations to improve the model for everyone else operating in the network.
Here are some strategies we’ve seen for incentivizing active human engagement with co-pilots:
- Create accountability: Ironclad, which offers an AI co-pilot to assist with legal contract drafting and negotiation, requires users to double confirm particularly sensitive AI suggestions. They’ve also experimented with requiring lawyers using the product to input their Bar Number.
- Give hints, not answers: Textio’s co-pilot highlights phrases in HR writing and then gives the user suggestions on how to change them to achieve their goal (e.g., attracting more diverse applicants). Periodically, Textio’s UX will highlight a phrase to change but not give a suggestion on how to change it, incentivizing the user to add their own ideas. Relatedly, TopHat, an ed-tech company, offers a co-pilot for students that offers hints as they do their work instead of answers.
- Align incentives: As users interact with co-pilots and add their ideas to the AI-generated suggestions, consider compensating users for their additions which drive business impact. If, for example, a sales rep modifies an AI suggestion that drives higher deal close rates across the network of users, you could pay them a commission on those deals.
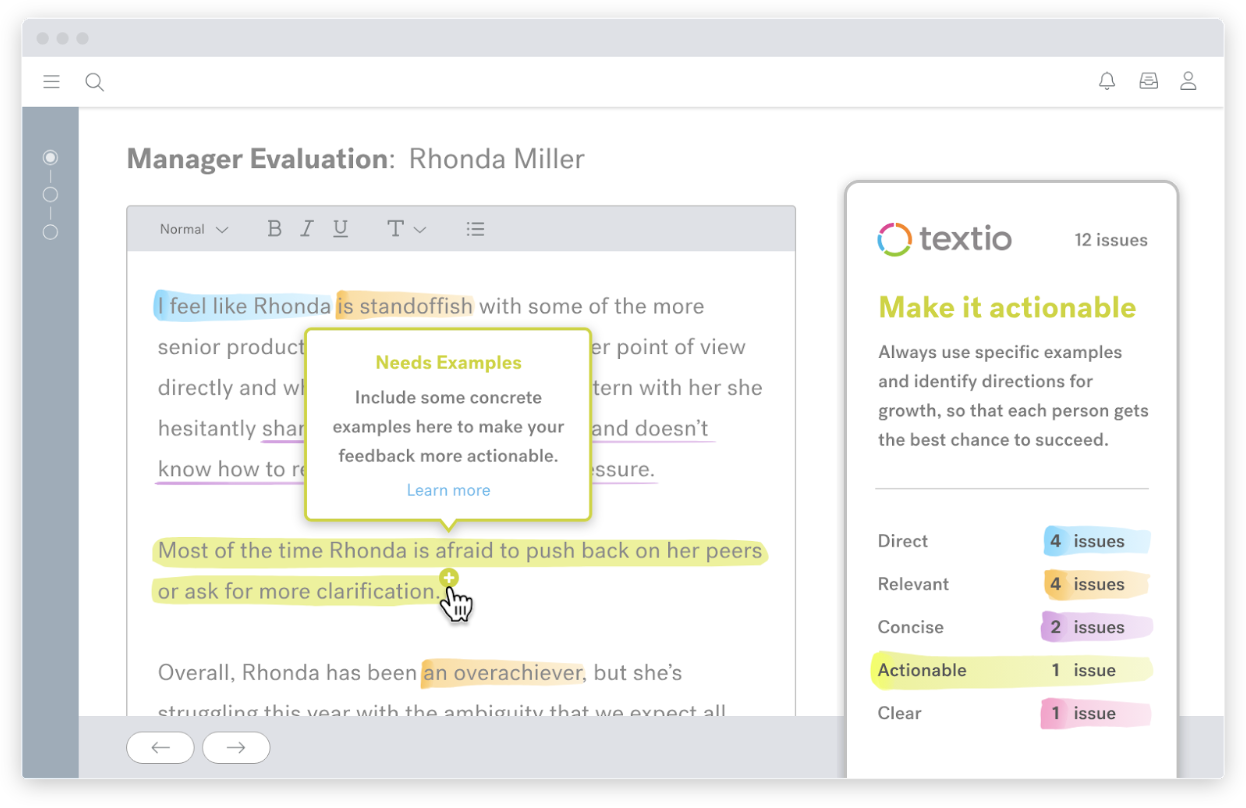
AI-enabled features: The menu
Depending on your product, there’s any number of AI-enabled features you could explore. Notion has done a nice job of surfacing an entire list of possibilities for users, like having AI write a blog post, author a poem, shorten or summarize a document, or translate text into a bevy of other languages. But it didn’t arrive at this list overnight.
What we heard from our panel of UX leaders is that utilizing chat and co-pilot interfaces helped them get to the best list of AI-enabled features right for their products.
Running with our Notion example, they discovered that more people were using the translation option than turning rough notes into a blog post. General chat is helping them every day to determine which AI features to prioritize in their roadmap. And now they can make these calls faster than ever before with more data — pointing to new methods for UX research in general.
Once you’ve surfaced the top ~10 AI use cases from your general chat interface, or you’ve found what users do the most with your co-pilot, you can pursue more features people actually need. And perhaps even more importantly, clearly message them in your product.
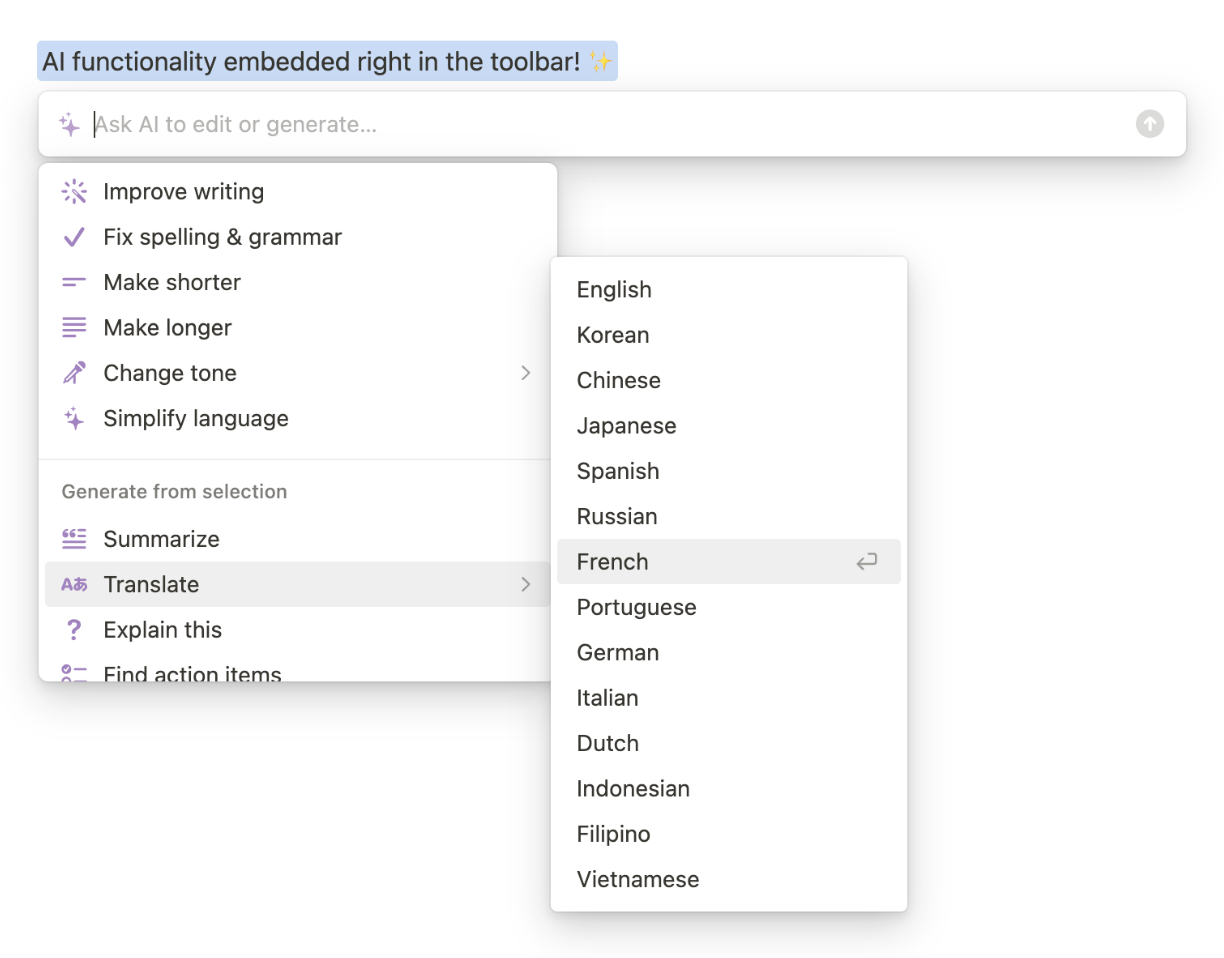
Button and toggle features, similar to Notion’s “make this sound professional” button, are very intuitive and say exactly what they do.
Unsurprisingly, these types of specific AI-enabled features exist all the way on the other end of the spectrum from general chat. They are the most direct, but also the most inflexible, producing predictable, narrow outcomes.
The success of these features depends entirely on the context in which you surface them. Some key tips for embedded AI features:
- Add AI to common menus. Notion added in its menu of AI abilities to its existing toolbar, even giving it a pithy keyboard shortcut like most of its other functionality. This puts AI in users’ pathways without having to go out of their way to use it. And the scrolling list of options makes it feel unbounded even though it is.
- Find proactive opportunities to highlight features. Know which actions or outcomes in your product would benefit the most from AI enhancement. When a user heads toward one of these, proactively call out the ability to use AI with a tooltip or pop-up so that they start building muscle memory around when AI is the right tool, and they feel their capability (and dopamine) boosted.
- Refine and prune. Actively track which features are being used. Don’t add new features without critically evaluating which existing features should stay. Don’t get bogged down by bloat. Fewer is often better.

The start of a conversation
Generative AI is, of course, rapidly evolving. And so are its UX deployments in SaaS applications. Countless important questions are still being answered, including how to build UX to maximize trust, given AI’s tendency to hallucinate.
We’re hoping to continue to create an ongoing conversation with builders across the ecosystem to share learnings as we go. To that end, we’d love to hear from folks who are actively deploying AI in their UXs. What in this piece resonated? What didn’t? Other important learnings you’ve had thus far? Reach out to us at jake@emcap.com and jessica@emcap.com. If you want to stay in the loop about future events, please fill out this form.
Thank you to all of the product and design leaders we spoke to for this piece, we wanted to acknowledge them here: Mike Wheeler (G2), Josh Abrams (Ironclad), Rick Nucci (Guru), Kieran Snyder (Textio), Jo Widawski (Maze), Jamie Cuffe (Retool), Linus Lee (Notion), James Cham (Bloomberg Beta), and Justin Cranshaw (Maestro AI).
Enjoying this article?
Sign up to gain access to our thought leadership and have future articles delivered directly to your email.