Cutting Through the Noise to Build an Enduring AI-Enabled Business
In February, we shared our thoughts on the current limitations of generative AI and why the technology is not yet tuned for B2B. Despite these limitations, we believe we’re in the early innings of a transformative era for B2B applications leveraging generative AI.
Founders face a daunting number of questions when thinking through how to capitalize on this opportunity. In recent conversations, the most common questions have been:
- What should founders build with this new generative AI tech?
- What generative AI-enabled business will be the most defensible?
- How can SaaS founders mitigate the risk of a bad AI trip?
Below are some of our initial thoughts. We’re learning alongside our portfolio founders and the broader SaaS community and are eager for reactions, rejections, and additions to these initial perspectives.
What should founders build with this new generative AI tech?
The excitement (and borderline hysteria) over generative AI has caused founders to lose sight of the most fundamental principle of company building: build something people desperately need. Much of the current startup market looks like a hoard of generative AI-enabled hammers searching for a nail.
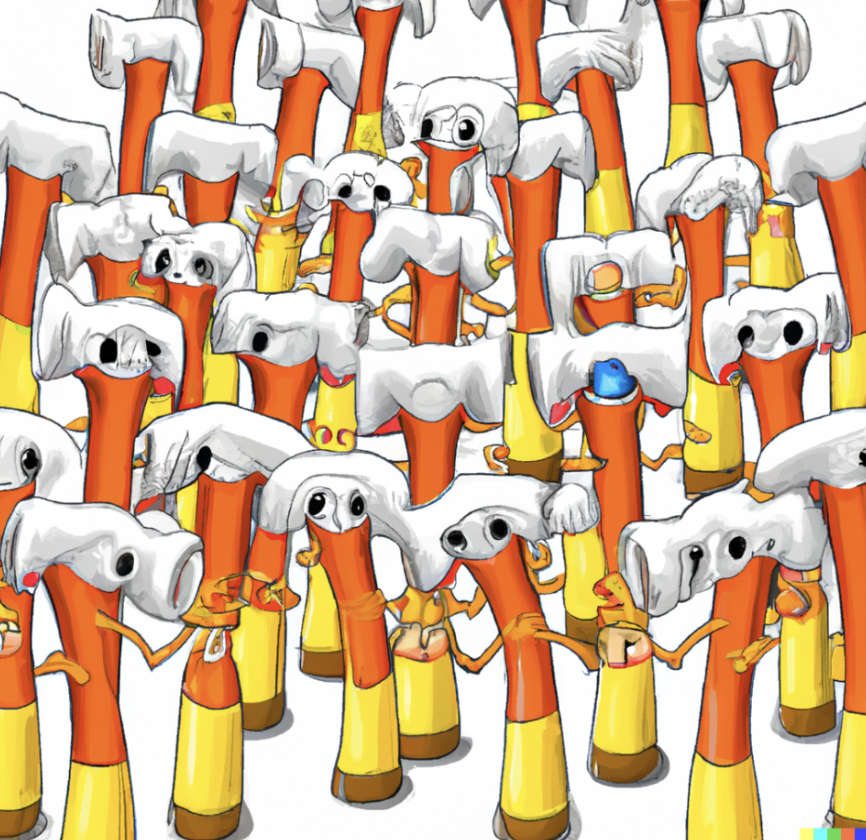
For new founders, start by looking for a neglected nail. You can find this nail from lived experience; Assembled and Regal were both started by former operators who tried and failed to solve their pain points with off-the-shelf solutions. Eric Yuan started Zoom after running WebEx’s Engineering team and deeply understanding the incumbent’s weaknesses. Or you can find this neglected nail from outside in via deep customer research.
Regardless, drive to find a unique insight. In a market deluged with “me too” generative AI-enabled solutions, the winners will be those that provide a unique solution to a desperate need (read more about achieving product-market fit here). Many of these needs will not have been addressable without GenAI, which is a strong justification for the current hysteria. But don’t let the fancy new tech tail wag the dog. Problem first, tech second.
For leaders of established companies, start by looking at your existing product roadmap (ideally derived with a similar “pain point hunting” approach). Identify which of these can be accelerated with recent generative AI tech and start building. The API format of products like GPT-4 means incumbents can integrate this tech relatively easily (see Doximity’s DocsGPT helping doctors draft letters to insurers and Ironclad’s AI Assist coaching people on how to redline contracts).
What generative AI-enabled SaaS businesses are likely to be defensible over time?
Given this relative ease of integration, how should founders think about defensibility? Certainly, as is always true, speed of execution matters. However, given that most of these businesses are building off of the same underlying off-the-shelf foundational models, differentiating on the AI itself will be tough, at least in the near term.
So, how do you know if you’re building a cool app on top of OpenAI vs an enduring business? Start by asking a simple question: “How much of the job to be done (JTBD) we’re focusing on can be done within OpenAI/Anthropic/etc?” Enduring businesses tackling complex jobs to be done likely require meaningful software beyond the generative AI element; the good old fashioned building blocks of SaaS, like complex workflows, data integrations, advanced permissioning, etc will likely prove to be the scaffolding around which defensible generative AI-enabled businesses are built.
Ironclad is an example of this approach. The company provides a complete digital contracting solution, allowing companies to draft, negotiate, and sign binding contracts. There is a tremendous amount of code required to pull this off, including a native text editor, robust permissioning, and tons of integrations. By adding AI to help with redlining, they are making it easier to complete the JTBD, but there remain countless parts of the JTBD that must be addressed with high-quality SaaS workflow.
Proprietary data will also play a role in defensibility over time, though the most valuable forms of it will likely come from workflows themselves. Data on the outcomes of key business processes (e.g., how quickly did the contract close) will be core to fine-tuning models for domain-specific use cases.
How can SaaS founders mitigate the risk of a bad AI trip?
In most B2B contexts, being mostly right isn’t good enough. If the wrong clause is added to a contract, lots of money (and jobs) are at stake. Given generative AI’s tendency to hallucinate, we think the best deployment approach in most B2B contexts is a co-pilot or coaching-style machine/human pairing. Think of this like an individual contributor (IC) to manager relationship (thanks to James Cham for the analogy). While the IC may produce a first draft, the manager is ultimately responsible for the outcome of the work. An AI coach should be thought of the same way- as a way for a worker to learn and accelerate but accountability must remain with the worker.
The key to doing this well in your application will be developing UX’s that encourage people to contribute actively rather than passively. This will ensure accuracy and provide creativity and mutations to improve the model for everyone else operating in the network.

See more of our thinking on how to build these “Coaching Networks” here. We’ll be diving deeper into each of these questions in future newsletters and on our Thoughts page. In the meantime, we’d love to hear from you if you’re building in the space. Please reach out to us at gordon@emcap.com or jake@emcap.com
Enjoying this article?
Sign up to gain access to our thought leadership and have future articles delivered directly to your email.