Artificial Intelligence isn't going to steal your job. It's going to save it.
We believe the future of work will be defined by Coaching Networks – AI learning loops that continuously identify specific, hyper-effective strategies from the brilliant outliers among us and distribute the best performing advice to the rest of the network.
Instead of fearing impending automation, we should embrace these digital coaching platforms as they help train the next generation of white and blue-collar workers to be more effective at their jobs.
AI headlines cast an unnerving shadow over our future.
As evangelists race ahead with claims of AIs that can diagnose patients better than seasoned physicians, file lawsuits more efficiently than cunning lawyers, and identify better leads than expert salespeople, some businesses cheer. Workforces shudder.

Some companies come out ahead in this dystopia, either productizing this new AI or quickly adopting the new technology to cut costs. Other companies disappear into bankruptcy. For workers, the picture is less clear. Layoffs. Unemployment. Automation. Obsoletion. There's no good ending.
The AI future you've been hearing about is wrong. It's cynicism, naivety, and fear-mongering wrapped into one.
— Gordon Ritter
This bleak future isn’t realistic. It misses one fundamental truth: humans are (and will be for the foreseeable future) better than even the most powerful computer systems in two critical areas:
- Understanding context.
- Humans can seamlessly understand context dynamically using a lifetime’s worth of unstructured data, especially when working closely with others in high-impact roles.
- Creativity.
- Humans can creatively solve problems in ways that computers cannot. Our intuition, and ability to make broad connections between ideas, is more powerful than we give ourselves credit for.
There's a better future on the horizon. Instead of using AI to replace humans, the next generation of AI will work in tandem with doctors, lawyers, retail employees, data scientists, writers, salespeople, and workers everywhere to learn from and help everyone do their jobs better.
We call these systems Coaching Networks.
Coaching Networks use machine learning – the backbone of today’s AI – to guide workers toward doing their jobs more effectively, finding the best strategies from the distributed network of similar workers using the software.
The software acts as a real-time, on-the-job coach, guiding employees to successful outcomes and gathering new creative behaviors that are fed back into the system. Rather than dispensing one-size-fits-all advice, Coaching Networks offer advice tailored to each worker and the task they’re doing.
We believe Coaching Networks will augment workers performing dynamic, high value tasks across the workforce while lower value, more static tasks will likely be automated away.
1. What processes will AI automate?
While productized AI won’t obsolete all of our jobs, there are certain processes that we’ll be able to automate away, freeing up valuable time and resources to focus on the parts of our jobs that matter. We call these Static Processes.
These soon-to-be automated Static Processes are where:
- There’s a limited, static set of inputs needed to determine outputs.
The data required to get an output is limited and immutable. As a simple example, you only need to look at a tomato’s color to determine if it is ripe. There isn’t a long list of contextual contingencies to check for and that won’t change over time.
- The associated rewards are linear, not exponential.
Even the best approach doesn’t drive massive rewards. The most effective tomato sorter in the world might only perform 20 or 30% more effectively than someone who just began.
- The process is (mostly) object-centric.
You’re largely working with things or machines, as these are typically the most objective and least dynamic processes. Tomatoes can’t decide to color themselves blue, thankfully.
These Static Processes are everywhere, dragging us away from the highly impactful, creative work that we’re more adept to perform. Data entry, transcription, even reading some types of MRIs and X-ray scans are all Static Processes that will soon be automated, freeing up time for workers to focus on higher-impact parts of their job, like data analysis and face-to-face patient care.
This isn’t a new phenomenon. Highly-repetitive, physical Static Processes have existed (and been automated) for centuries. The printing press, the cotton gin, the tractor – all served to replace Static Processes – calligraphy, separating cotton fibers and seeds, tilling the ground – that had once been performed by mass populations of individuals.
The Robotic Process Automation (RPA) sector, as its name suggests uses AI to automate static tasks, is thriving. Automation Anywhere (started in ‘03) raised $550M, UI Path raised $408M, and Blue Path raised $59M, all to help businesses automate static processes like data entry that eat up millions of hours of productive work at large businesses with AI.
As sensor technologies advance, computers are better able to capture and process larger universes of input data and thus automate more complex static processes. Perhaps the static process that will have the biggest near-term impact on society once automated is driving: like Google’s Waymo, Apple, Amazon, Cruise, Uber, Tesla, and dozens more are close to announcing varying levels of autonomous cars.
2. What processes will humans keep doing even better with AI?
New intelligence models – Coaching Networks – are coming to help us find exponential returns in our work and save us from automation.

Coaching Networks attack Dynamic Processes – as opposed to Static Processes – where there is no objective best approach. These processes are where:
- There is an unbounded, dynamic set of inputs necessary to determine outputs.
There is a large set of ever-changing variables that affect your output. For example, when selling a customer on a product, there are thousands of variables affecting yours odds – the questions you ask, your tone, their background, how much of their budget is left, etc. As the way we communicate changes over time, the impact of these variables on the process changes as well.
- The associated rewards are exponential.
The best strategies drive massive rewards. The best salesperson in the world can sell an order of magnitude more product than the average rep. There’s no cap to their success. Outliers matter.
- The process is (mostly) human-centric.
You’re largely working with other people, as these are typically the least objective and most dynamic processes. Salespeople spend all day working with other humans, whose personalities and goals change every day based on thousands of variables.
These Dynamic Processes are everywhere. The perfect developer, writer, salesperson, or marketer doesn’t exist – instead, each of these professions is centered around a number of Dynamic Processes that attempt to solve high-value, fundamentally human problems. Rather than dispensing “one-size-fits-all” advice, Coaching Networks offer coaching that’s uniquely tailored to each worker and the task they’re doing at any given moment.
Humans should focus on dynamic processes that reward our creativity. We're biological beings that don't conform to digital patterns.
— Gordon Ritter
Instead of attempting to achieve an unrealistic goal – “We’re going to replace every writer with a perfect AI!” – Coaching Networks highlight the best strategies from the entire network and let each worker decide how to approach the problem. This lets workers combine the wisdom of the best among us with their own creativity, context, and brilliance to produce exponential returns.
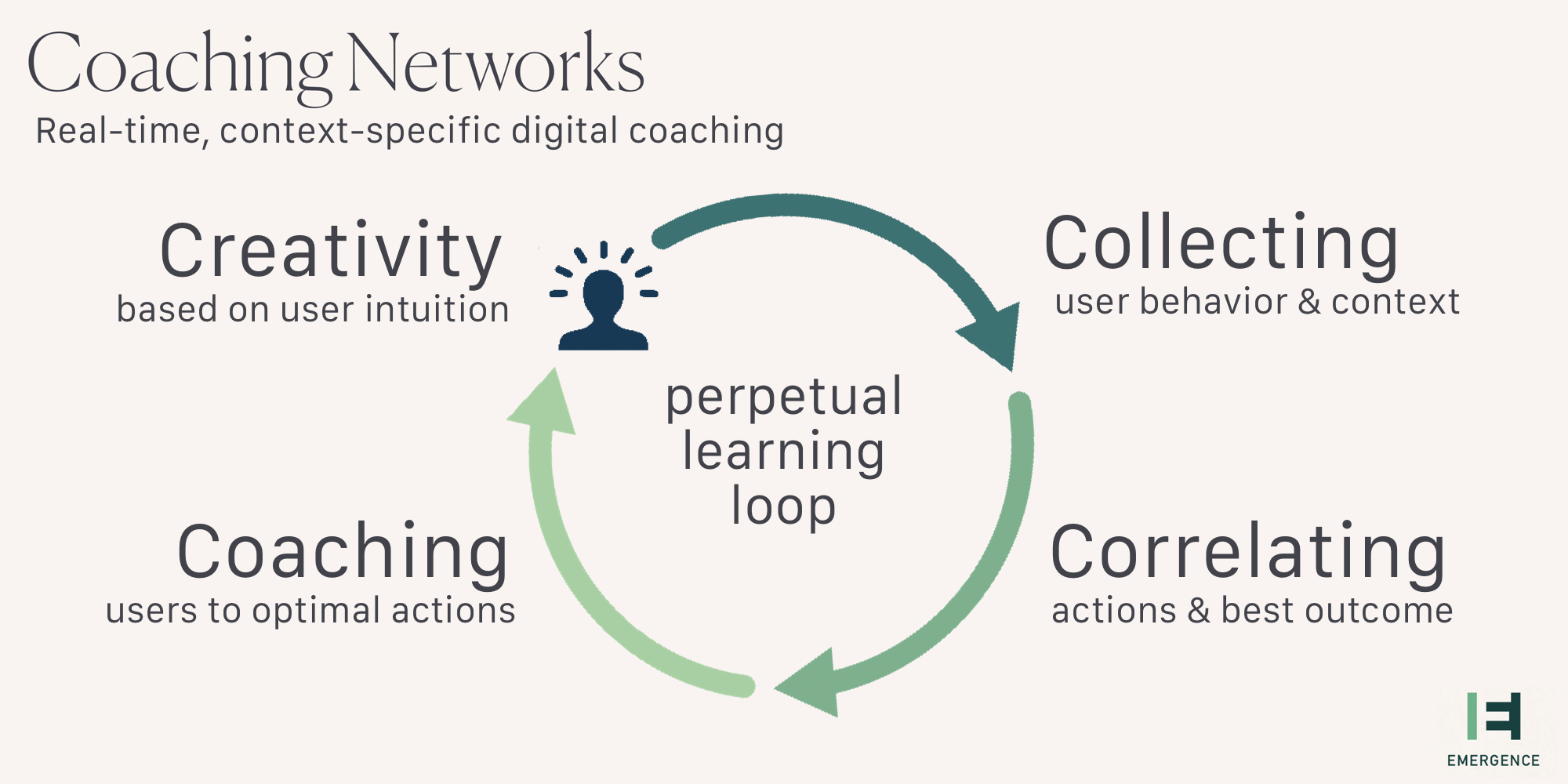
Here are the four steps each of these networks use:
Collecting user behavior & context
First, a range of sensors collect the user’s behavior – what decisions they made – and the specific context used to make the decision. These sensors can work through a variety of modals: your browser, Zoom, your phone, even your smartwatch and AirPods.
Let's take an imaginary Coaching Network: one designed to help engineers build software. In this case, the user behavior would be how the engineer actually decided to architect their code and the context would include: the parts of the system that were already built, which open source libraries the developer chose to use, the requirements of their current project, the intended user and frequency of use, ad infinitum. The list goes on.
The sensors also collect the end result of the behavior, typically tying into an existing system of record. In this case, the sensors could look at Jira to collect how long the project took to complete and whether each project satisfied the requirements laid out at the beginning of the project. It could even connect to a CRM system to see how well the software has been selling.
Correlating user action & best outcomes
Next, the AI compares the individual user’s behaviors to outcomes to identify best practices and brilliant outliers in the network.
In our case, the AI would identify situations where developers had built a specific program that performed extraordinarily well on the dimensions above. Crucially, it will identify the key granular contextual elements in which that developer was an outlier.
Coaching users to the optimal actions
Next, the Coaching Network – through a highly-tuned, integrated UI – delivers advice to users based on what it believes to the optimal strategy within each specific context.
In our Coaching Network for software developers, this could take the form of suggested pre-made code snippets served up to developers in-line and in context, or real time feedback that there might be a simpler sub-routine available that achieves the same goal.
Human creativity based on intuition.
This is the critical step. After users receive advice, they decide to implement the advice, modify it, or ignore it in favor of their own intuition.
As users interpret and actively decide what to do, the AI watches to see which path is most successful at driving business outcomes. As outliers and contrarians reject these recommendations, their creative brilliance quickly spreads throughout the network. The network advances because we are all different and have unique perspectives.
In our Coaching Network for software developers, this would take the form of an engineer ignoring the suggested code blocks and writing new code from scratch, or editing the suggested code block to fit their specific goal.
The best Coaching Network UIs will, in addition to making suggestions, encourage users to “mutate” the recommendations by deploying their own creativity.
A perpetual learning loop
This loop – from AI recommendation to user action, to result, to improving the AI – continues in perpetuity as users engage with the system.
Rather than requiring larger and larger training datasets, which can quickly become expensive or logistically unfeasible, Coaching Network AIs improve perpetually as more users engage with the system by creating a data flywheel. Just like any social network, which becomes more valuable as more users interact with it, Coaching Networks become more adept and helpful as more users engage with the system.
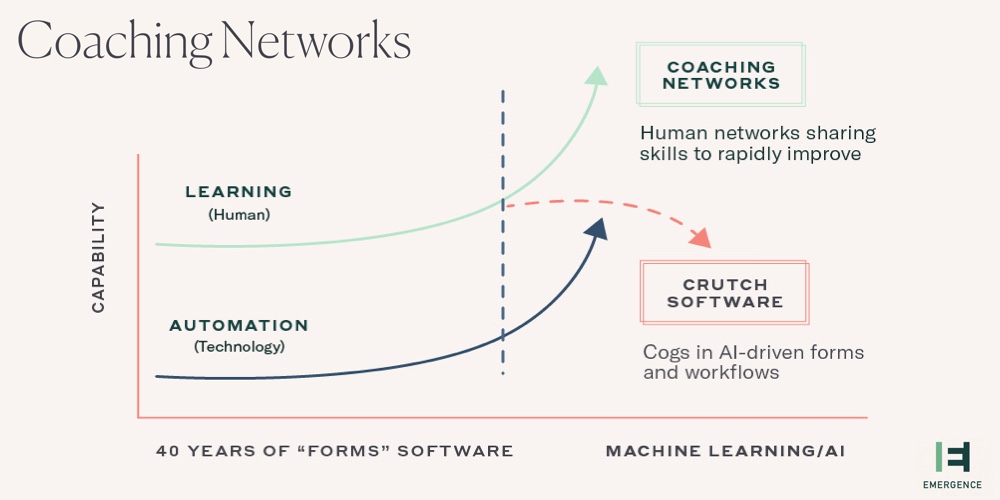
Humans are the only mutation engine in the age of AI. We are the core of a perpetual algorithm that’s more dynamic than software will ever be.
— Gordon Ritter
3. Why we're primed to adopt Coaching Networks
We've seen the shift from on-prem to the cloud mint dozens of enterprise unicorns with our investments in Salesforce, Veeva, Box, Yammer, Zoom, SuccessFactors, and more.
The next shift in enterprise will organize around coaching networks, minting unicorns in a race to coach the world’s workers to be their best selves.
As we improve our ability to build AI, we can either treat it as a crutch that relieves us from thinking — like Waze or Google Maps — or as an asset that helps us use our brains more completely, effectively and creatively.
4. How to kickstart a Coaching Network
Building a Coaching Network from scratch is more involved than quickly labeling a dataset.
First, you need to define a focus for your AI. Make sure the task you're looking to augment is domain-focused, as data unrelated to the specific problem isn't helpful.
For example, Textio – one of our portfolio companies – is designing a Coaching Network to help people write better. Instead of defining the entirety of writing as their focus initially, they focused on helping people write effective job descriptions. Brevity is the soul of wit... and focus is the soul of a Coaching Network.
Second, you need to build a proprietary dataset for your focus.
Not all datasets are not created equal.
To fuel a Coaching Network, your dataset must be deep, dynamic, and proprietary. This holy trinity of characteristics is the fuel that can turn empty, commoditized workflow software into rich, defensible recommendation engines.
Every time a user engages with your product, you have the opportunity to collect data from the interaction that systematically make the product even better for every user in the network.
— Jake Saper
Very few companies start with this user-driven flywheel. They start with hacks. There are four main ways to begin hacking your way into a proprietary dataset:
- Scraping.
Aggregating publicly available but scattered data. This can be cheap and easy to start, but is easily copied. Corelogic is perhaps the best example of what can be built on the back of offline scraping; they collect public records data from government offices across the country and sell the packaged data to real estate players for large sums.
- Partnering.
Working with established entities that don't have the bandwidth to leverage their large unstructured datasets. This can quickly scale your closed loop system but requires the right partner and partnership structure. Tractable, which provides an AI that improves car accident repair processes, is a good example of a startup that has hacked its way into success by partnering with industry incumbents.
- Crowdsourcing.
Aggregating individual answers or data points from teams or workers, just like Amazon’s Mechanical Turk. While this often gives you more control over the data formatting, it can be hard to scale and easy to replicate.
- Workflow-first.
A two-step method beginning with building workflow services, driving usage via the workflow, and then looking for ways to make use of the data captured. This is quickly monetized, but most businesses don't make it to a two-step workflow for a variety of reasons. EmCap portfolio company Guru’s Coaching Network was built on top of their knowledge management workflow software.
Coaching Networks, humanity’s edge to win the race against automation.
— Gordon Ritter
5. Requests for Coaching Networks
Many of the best Coaching Networks we’ve funded were ones that surprised us, not ones we were waiting for.
At the same time, there are a number of verticals that we believe are primed for a revolution with a Coaching Network. Working on anything in this space? Reach out. We’d love to chat.
- Software development.
Much of coding new software is rote – integrating frameworks, libraries, and tools that have already been built or fixing common bugs that have plagued thousands of engineers before.
There are dozens of approaches one could take to building coaches for both backend and frontend engineers, especially given the staggering number of niche programming languages, frameworks, and tools.
- Lawyers and paralegals.
The vast majority of “practicing law” is relatively tedious, scanning through reams of contracts or cases for particular phrases or terms. It’s difficult to synthesize exactly what to look for in a simple search query, but lawyers “know it when they see it.”
We’re excited to see teams build in this space, democratizing access to legal help. When lawyers and paralegals alike do their jobs more effectively, it brings the cost of routine legal work down and helps more people assert their rights.
- Product management.
Managing large engineering, design, and product teams towards deadlines and KPIs will never be automated. The most successful PMs blend EQ, IQ, and deep technical perspectives to lead entire teams towards success.
These visionary PMs are often eager to dispense advice, but finding the right advice for each complex and unique situation is difficult. Similarly, performing user research – surveys, interviews, focus groups – requires deep product insights and high EQ to know when to push respondents for more information or highlight seemingly banal responses.
We’re excited to see Coaching Networks help bridge the gap, transferring valuable, context-specific insights between PMs in need.
- Sales prospecting.
Sales Development Representatives spend much of their days on routine work, qualifying prospects based on a set of tangible and intangible characteristics. We’re excited to see companies use Coaching Networks to make SDRs job more efficient and more productive.
Given the nature of the data collected, startups in this space focusing on one industry will have the unique opportunity to expand into other industries quickly or up into coaching Account Executives to close prospects more efficiently.
- Invoice collection.
Billions of dollars of commerce rely on outdated invoices, paid slowly and manually by most companies. This is a massive problem for small, cash-strapped companies: if a company with $6M in revenue can bring its accounts receivable days outstanding – how long it takes someone to pay you – from 90 to 60 days, they’ll get an extra $500K in cash on hand every month.
There are reams of data surrounding emails, calls, and strategies used to successfully collect cash from a customer. We’re excited to see companies help finance teams more efficiently and effectively collection payments.
- Interviewing.
Asking the right questions during an interview – asking candidates to reveal their weaknesses and true intentions while attempting to convince them to join your company – is as much an art as skill.
We’re excited to see companies build Coaching Networks for interviews. One model could optimize for highlighting positive or negative signals from the candidate based on conversion (how likely the candidate is to accepting an offer) or, more importantly, performance (how well the candidate performs as an employee post-hire). As with any skill-assessment AI, these types of networks can be fraught with systemic bias.
A second model exists to solely help interviewers give better interviews, offering real-time suggestions proven to better conversion or performance post-hire.
- Content marketing.
Coaching Networks thrive when a percentage of a population has unique insights or creative perspectives to approaching a problem. Content marketing is a natural fit, with a small cohort of effective writers effectively leading the pack.
As companies increasingly compete for SEO rankings and traffic, there is a unique opportunity to help facilitate that transfer of information, helping everybody improve in the process.
- Medical professionals.
Medical professionals are trained to optimize for every case, but most focus on treating the expected cases that appear at a disproportionately large rate.
Doctors and nurses that work hand-in-hand with a Coaching Network will be more effective than either team working alone. With millions of incorrect diagnoses every year, we can’t wait to see companies materially improve and save lives with AI.
- Managers.
“People don’t leave companies, they leave managers.” A great manager is key to retaining employees, yet most management training lacks context required to be effective.
While admittedly difficult to implement, we’re excited to see Coaching Networks embed themselves in organizations – in Slack channels, 1-on-1’s - to facilitate feedback and communication, optimizing for employee retention and happiness. Start in a highly focused domain in this area (e.g. meeting agendas and follow-ups) and nail that use first to start your network.
- Maintenance, plumbing, electrical work.
The best plumbers, electricians, and other maintenance workers have developed their own strategies and intuitions for dealing with problems of all sizes.
As cameras shrink and Augmented Reality glasses promise to embed themselves in our lives, there will be a day where workers from every vertical receive real-time, context-sensitive suggestions designed to optimize for cost, speed, and safety.
- Security and data privacy.
Consumers win when companies work together to protect data. Today, most infosec professionals at various companies already work together to fix vulnerabilities.
We’re excited for the future of collaborative security, powered by Coaching Networks that automatically suggest patches, architecture changes, or other strategies to protect consumer’s data and privacy based on threat models identified by other companies or security teams.
- Data science & analysis.
Tools like Looker, Periscope, and EmCap portfolio company LogDNA make it easy for data scientists to run queries on large datasets – knowing which query to run requires years of training and context.
An individual query and subsequent results can have outsized impacts on the operations of a business. We’re excited to see Coaching Networks help data scientists do their jobs more effectively, suggesting queries or reports in real-time based on types of previous queries that have been used or referenced heavily.